User-led algorithmic auditing with IndieLabel
The first public-facing deployment of IndieLabel, a web app that enables everyday users to perform algorithmic audits, is now live!
Prompt transformation and the Gemini debacle
A term I recently learned, “prompt transformation”, describes how prompts are manipulated under the hood to provide more diverse results.
A call for better access to AI systems for independent testing
An open letter calling for AI companies to make policy changes enabling to protect good faith independent testing of their products.
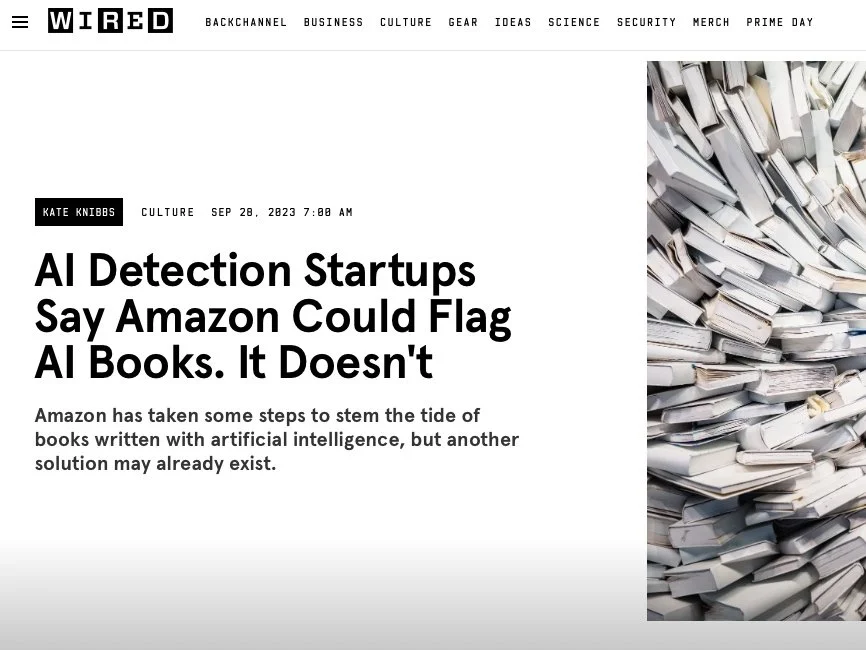
Wired falls for bogus claims of AI writing detector startups
Wired is pushing the false idea that AI writing detectors actually work.
New developments with AI writing detectors (they still don’t work!)
New evidence keeps surfacing that AI writing detectors don’t work reliably.
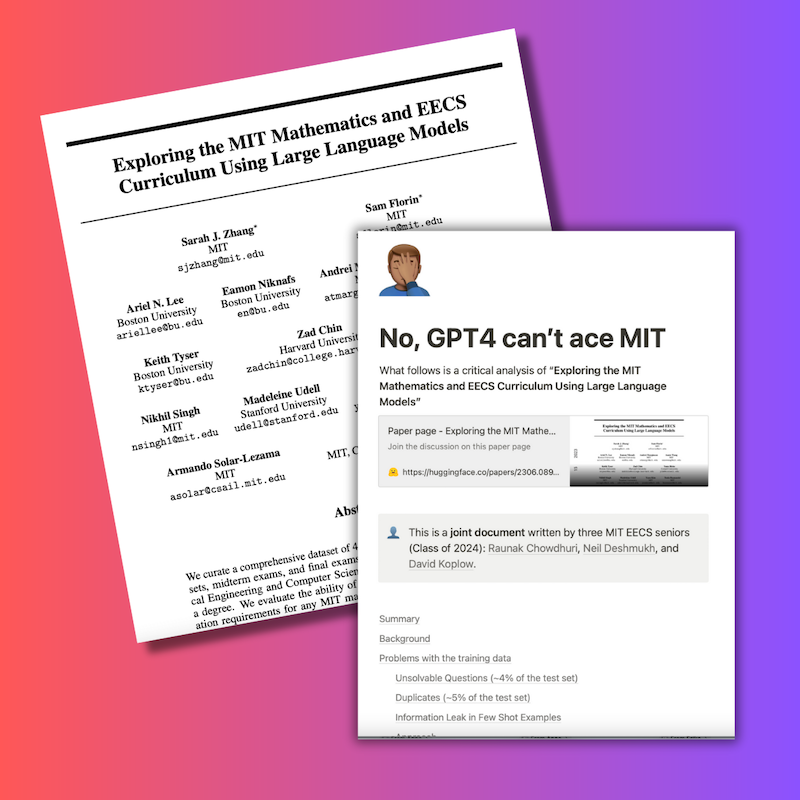
Latest overblown AI claim: GPT-4 achieves a perfect score on questions representing the entire MIT Math, EE, and CS curriculum
The paper, released on arXiv two days ago, is getting a lot of attention. Some issues with it were immediately apparent. Then three MIT students dug up the study’s test set, and discovered things were much worse than they initially appeared.
Hype alert: new AI writing detector claims 99% accuracy
Multiple media outlets are reporting on a recent study published by University of Kansas researchers. Per these reports, the KU researchers have achieved near-perfect accuracy at detecting AI use in “scientific writing” or “academic writing.” But let’s take a look at what this study actually did.
Should we have a government-funded “public option” for AI?
My reaction to the article “How Artificial Intelligence Can Aid Democracy” by Bruce Schneier, Henry Farrell, and Nathan E. Sanders, published April 21, 2023 by Slate.
Guardrails on large language models, part 4: content moderation
The final post in a four-part series on the guardrails on large language models.
Guardrails on large language models, part 3: prompt design
The third in a four-part series of posts about the guardrails on large language models.
Guardrails on large language models, part 2: model fine tuning
Prompted by Bing/Sydney’s zany behavior, this series of posts gives a non-technical introduction to the guardrails on large language models (LLMs).
Guardrails on large language models, part 1: dataset preparation
With the recent spate of news about Bing/Sydney going haywire, I’ve noticed some misconceptions about the guardrails on large language models (LLMs). To help dispel some of them, in this series of posts I’ll give a non-technical introduction to each of the four major points of control for LLMs.
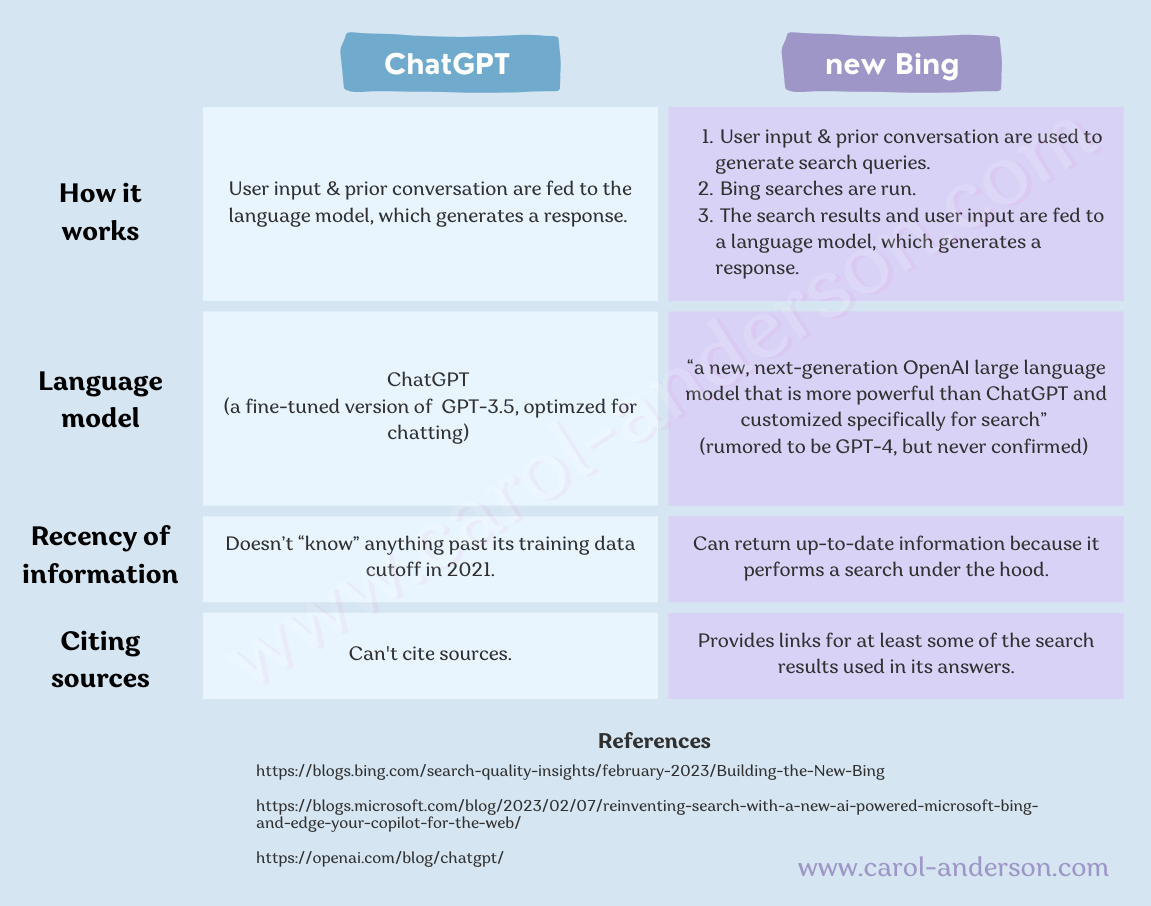
What’s the difference between the new Bing and ChatGPT?
Wondering how the new AI-powered Bing is different from ChatGPT?
I made this graphic to show their key differences.
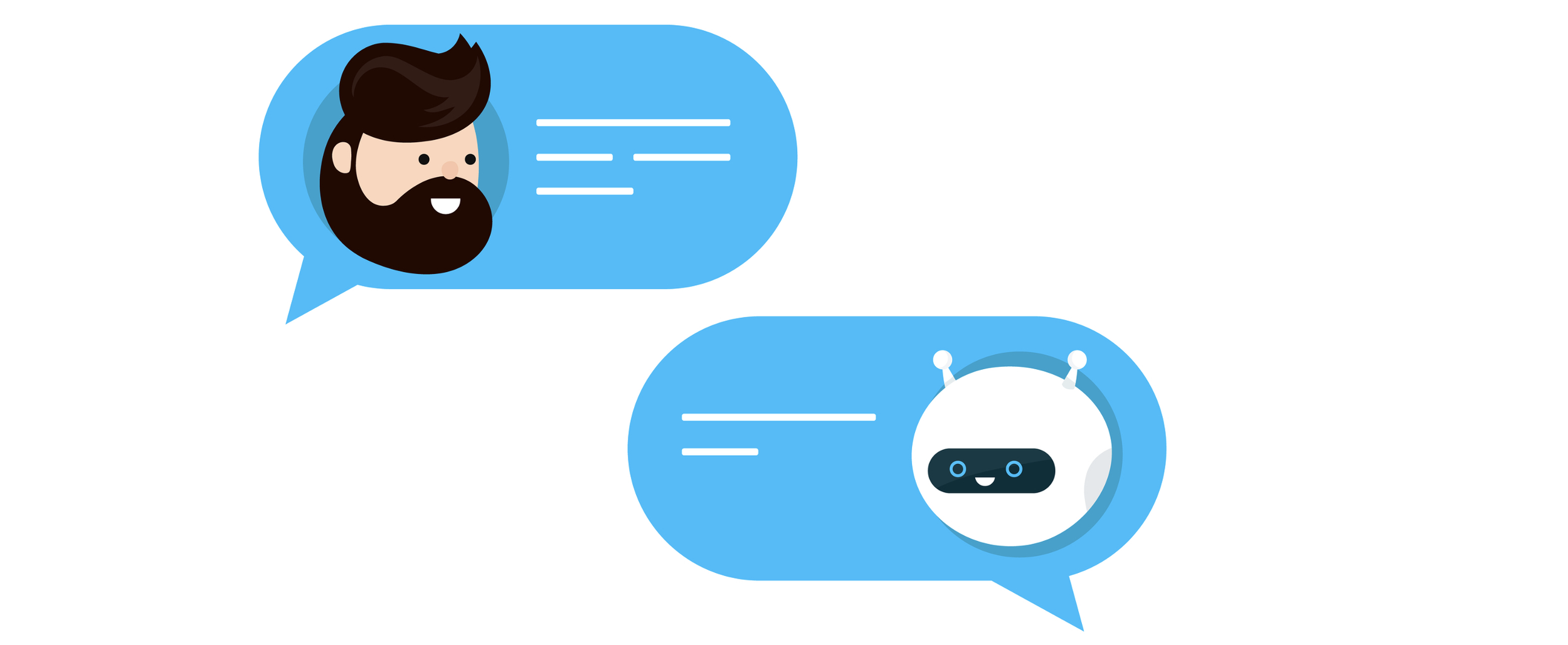
Koko, ChatGPT, and the outrage over corporate experimentation
Mental health service Koko sparked outrage by announcing it experimented with ChatGPT for mental health support, apparently without informing users. (It turned out users were informed all along, and the CEO’s Twitter thread was just really confusing.)
Here, I dig into the outrage and argue that much of it was focused on the wrong issue: the ethics of corporate experiments.
Why I don’t think ChatGPT is going to replace search anytime soon
There’s been a lot of breathless coverage of ChatGPT in the past week. One comment I keep seeing on social media is that it’s going to replace Google and other search engines. I don’t think that’s going to happen anytime soon, and here’s why.
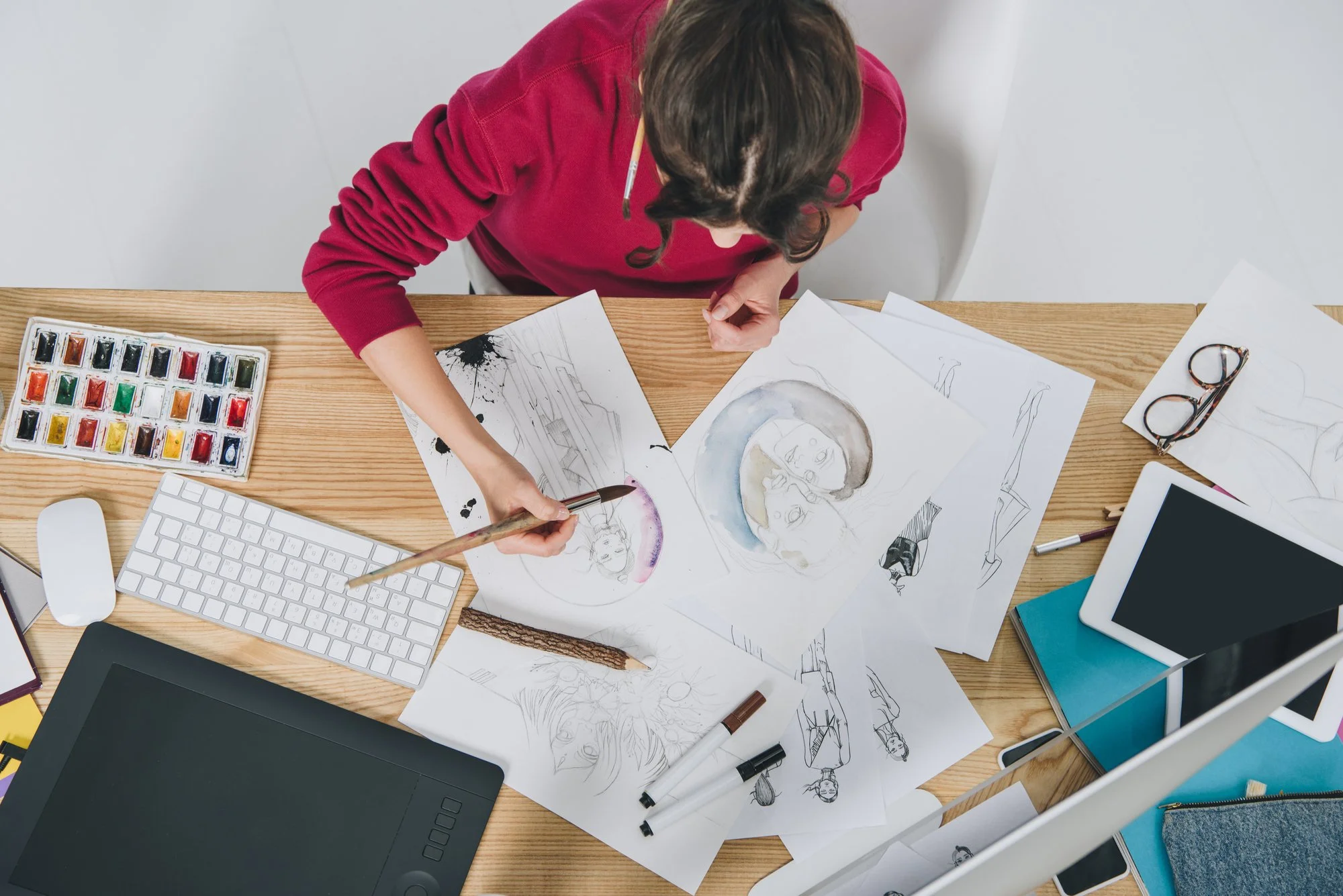
Do stock image creators know they're training AI to compete with them?
Recent announcements have revealed that stock image collections are being used to train generative AI. Compared to using web-scraped data, this is less legally risky and potentially more fair to the creators of the images. But I have some nagging concerns about it.
Class-action lawsuit filed over copyright and privacy issues stemming from GitHub Copilot
Last week I posted about the copyright and privacy risks associated with large language models. One of the examples I discussed was GitHub Copilot, the code-writing assistant based on OpenAI's Codex model. One of the key problems with Copilot relates to code licensing. Today, the issue headed to court.
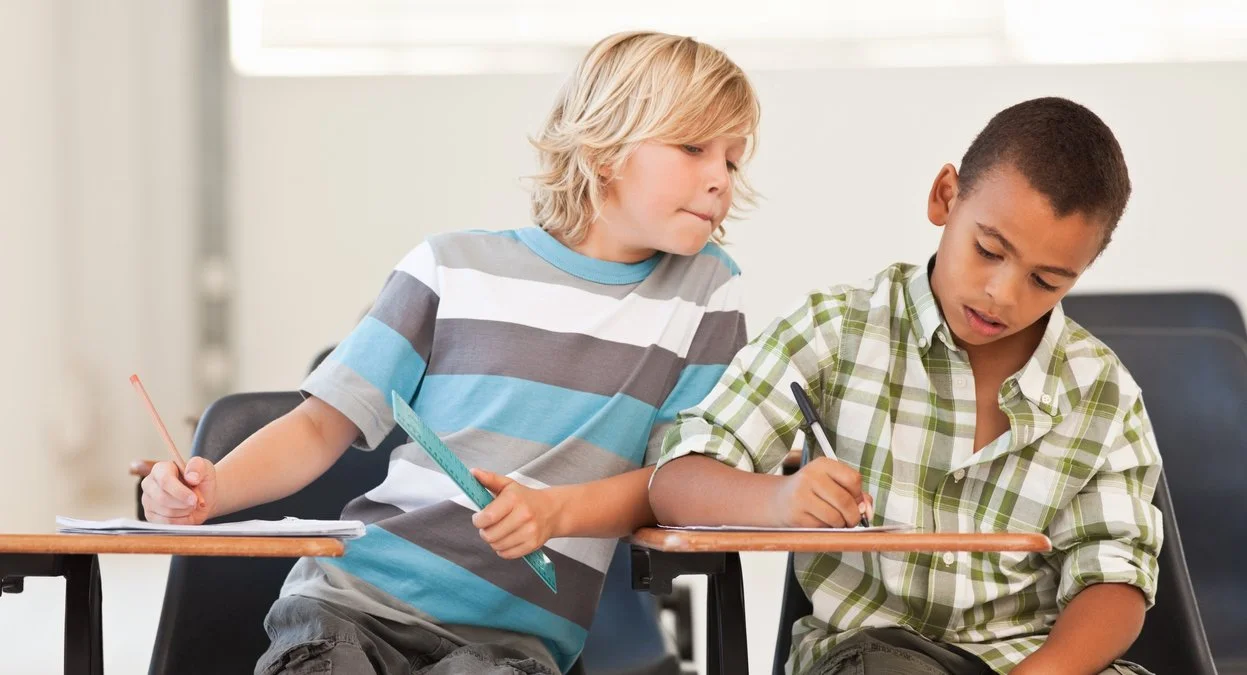
Large language models can steal work and spill secrets. Here’s why we should care.
Large language models are trained on massive datasets of web-scraped data. They memorize some of it, and can regurgitate it verbatim – including personal data and copyrighted material. Is that a problem?